共同研究者 藤田さん、M1真鍋さん、早矢仕講師がIEEE BigData2023にて研究発表を行いました。
2023年12月21日
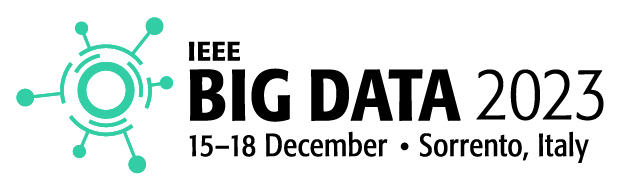
共同研究者 トヨタ自動車の藤田氏、早矢仕研究室 修士1年の真鍋さん、早矢仕講師が12月15日〜18日にイタリアのソレントで開催されたIEEE International Conference on Big Data 2023 (IEEE BigData2023)にて研究発表を行いました。
タイトル:Topic-Based Search: Dataset Search without Metadata and Users' Knowledge about Data
著者:Yukihisa Fujita, Teruaki Hayashi, and Masahiro Kuwahara
アブストラクト:With the advancement of information technologies, we can obtain various kinds of data, which can be leveraged for various purposes. The availability of a large amount of data is a desirable situation. However, it makes dataset retrieval a time-consuming and complex task. Conventional dataset search methods require unified metadata and knowledge about keywords representing the datasets. In other words, they require user knowledge regarding the datasets, such as the terms used in the dataset and fields in the metadata. To address this issue, we propose a topic-based search method without metadata, especially for users lacking knowledge about the datasets. The topic-based search can find datasets by using not the exact keywords but abstract keywords described as topics. In this paper, we focus on table data, which contain column names and data values and are widely used for storing data. As preliminary analysis, we collected and analyzed public datasets available in Japanese data portals to clarify the features of datasets that should be searched through dataset search. The analysis results revealed the use of many general and common keywords as column names, but it is difficult to implement a dataset search using only column names. Therefore, based on the analysis results, we decided to use embeddings converted from the datasets to utilize both column names and data values to extract topics from datasets. The experimental results showed that we can extract topics from datasets by using the topic modeling method and obtain better search results when compared with the search method using exact keywords.
本研究は、トヨタ自動車株式会社との共同研究の成果です。
タイトル:Variable-based Learning Considering Topic Specificity in Heterogeneous Data Clustering Tasks
著者:Kosuke Manabe, Yukihisa Fujita, Masahiro Kuwahara, and Teruaki Hayashi
アブストラクト:In recent years, data mining by interdisciplinary co-creation has been attracting social attention, and various types of data have been published for free or for a fee. Data publication encourages the exchange and combination of data between different institutions, which is helpful for interdisciplinary data collaboration. However, issues remain in data search to design high-quality data for interdisciplinary data discovery. Variables are frameworks for data and are thought to have reflected the topics of the data and the intent of the data design. This study aims to quantitatively investigate the relationships between data topics and variables for a large dataset and provide suggestions that contribute to data design and exploration. In this study, we quantitatively evaluated the probability of occurrence of variables and their pairs for each topic to determine a specific relationship between the topics and variables and applied the clustering considering them.
本研究は、トヨタ自動車株式会社との共同研究及び科研費基盤B研究「データ流通市場のダイナミクスの解明と制度設計(20H02384)」の支援を頂きました。
タイトル:Exploring the Fundamental Units of Semantic Representation of Data Using Heterogeneous Variable Network in Data
著者:Teruaki Hayashi, Yukihisa Fujita, and Masahiro Kuwahara
アブストラクト:The value creation achieved through the exchange, distribution, and collaboration of data among different organizations has garnered significant attention as a new source of innovation. The mathematical treatment of the meaning of data helps measure its "quality" to formulate evaluation criteria for data exchange between stakeholders with distinct background knowledge in data ecosystems. This study examines the structure of data morphemes, the fundamental units of semantic representation of data, by conducting network and association analyses of variables present in metadata from diverse fields. Network analysis identifies the globally sparse and locally dense characteristics of variable co-occurrence networks and highlights essential relationships and core variables. Key findings include the discovery of "depth," "sediment/rock," and "sample code/label" as both universal variables and crucial nodes between datasets used in the experiment. Association analysis reveals vital variable pairs, such as "age" and "ring width" or "latitude" and "longitude." This research may provide a understanding of the structure and meaningful representation of data, facilitating smooth data exchange and utilization practices among stakeholders with different domains, purposes of data use, and background knowledge in data ecosystems.
本研究は、トヨタ自動車株式会社との共同研究及び科研費基盤B研究「データ流通市場のダイナミクスの解明と制度設計(20H02384)」の支援を頂きました。
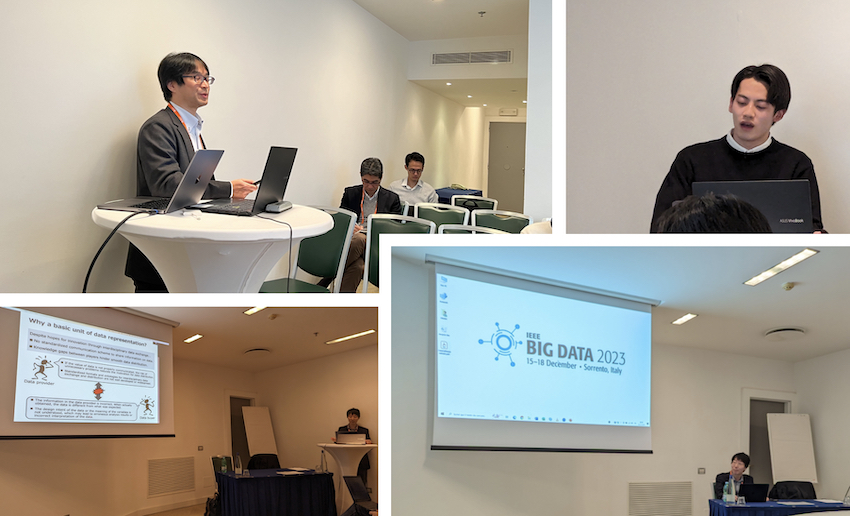