西尾さんがIEEE IEEMでポスター発表を行いました。
2024年12月18日
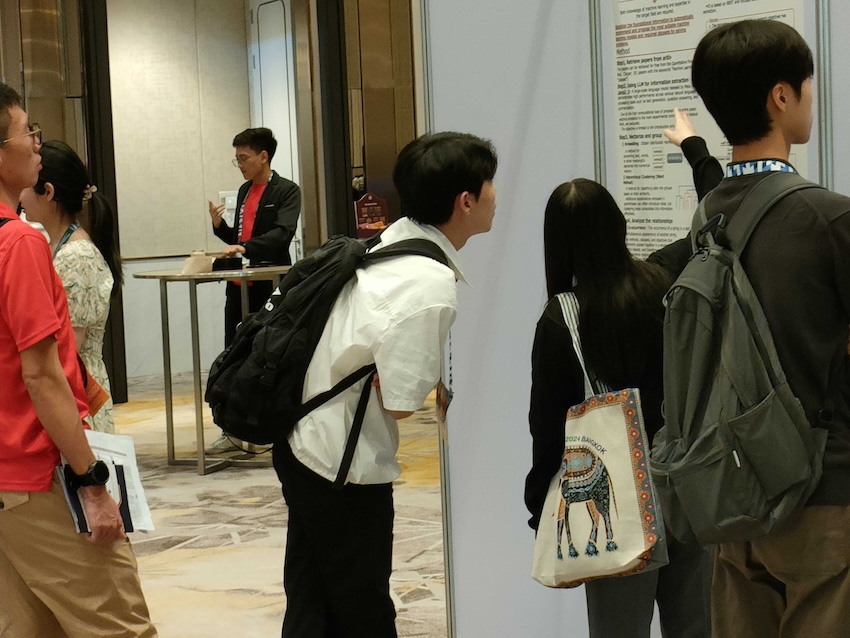
共同研究でご一緒している愛知工業大学 知識マイニング(野中)研究室の西尾さんがIEEE International Conference on Industrial Engineering and Engineering Management (IEEM)にてポスター発表を行いました。
本研究では、LLM(Llama3)と埋め込みモデルを活用して、学術論文から研究目的、ML手法、データセット名を抽出し、これらの相互関係をネットワーク分析で可視化する新たな方法を提案しました。このアプローチは、特に経済分野の論文で有効性が確認され、ESGデータを活用した研究への応用も示唆される結果を得ました。
タイトル:Extraction of Research Objectives, ML Model Names, and Dataset Names from Academic Papers and Analysis of Their Interrelationships Using LLM and Network Analysis
著者:S. Nishio, H. Nonaka, N. Tsuchiya, A. Migita, Y. Banno (Aichi Institute of Technology), T. Hayashi (The University of Tokyo), H. Sakaji (Hokkaido University), T. Sakumoto (Nagaoka University of Technology), K. Watabe (Saitama University)
アブストラクト:Machine learning (ML) has become a key tool in many sectors. Selecting the most suitable ML models and datasets for particular applications is essential to the successful use of ML in industry. Nonetheless, this process demands expertise in both the ML techniques and the specific an application domain, which can result in a steep learning curve. Consequently, research aimed at automatically extracting research goals, ML techniques, and dataset from academic literature is crucial for streamlining recommendations of appropriate methods. Traditional approaches for extracting information from literature have typically been confined to recognizing entities such as ML models. In response to this limitation, the current study introduces a novel approach that focuses on extracting tasks, ML methodologies, and dataset names from research papers, while also exploring the interconnections between them by leveraging large language models (LLMs), embedding techniques, and network clustering. The proposed approach, using Llama3, demonstrates strong performance, with an F- score above 0.8 in various categories, thereby confirming its effectiveness. Moreover, evaluations on research papers within the financial domain validate the utility of this method, presenting critical insights into the deployment of contemporary datasets, particularly those associated with ESG data.